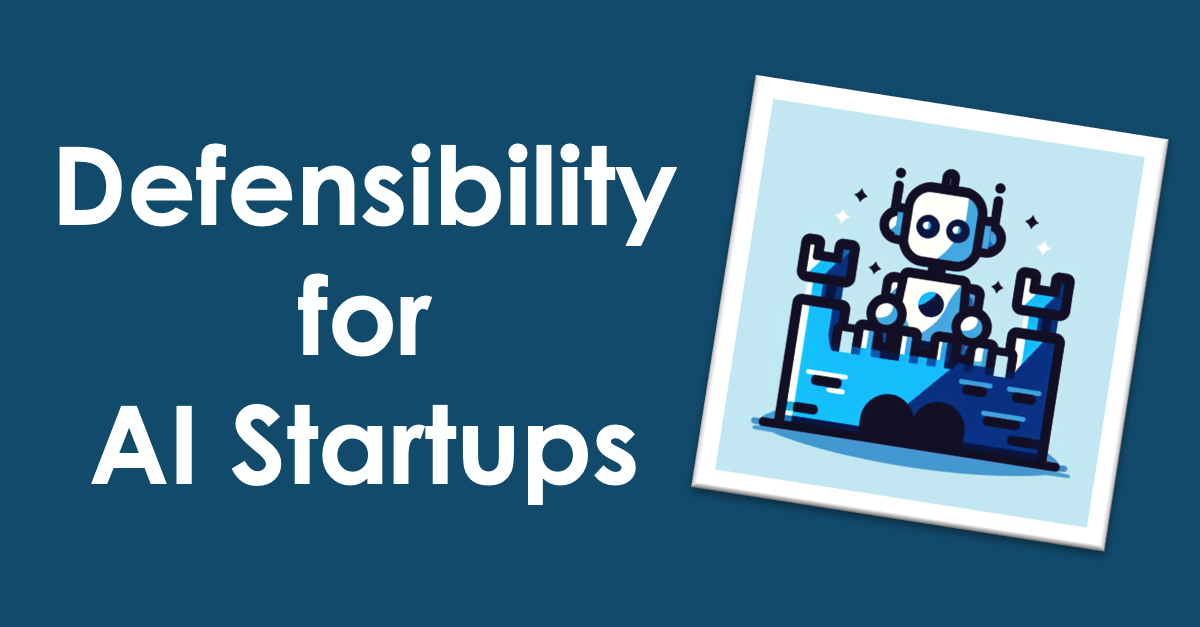
Defensibility for AI Startups
A significant portion of AI startups that have emerged during the last year are essentially thin wrappers around OpenAI’s API. This is the archetypal “ChatGPT for X” startup, where X can be anything from marathon planning to insurance claims handling.
The problem with building a business around a thin wrapper of another company’s API is that there is almost zero defensibility against copycats who create a clone of your product. Y Combinator is already funding numerous AI startups pursuing almost identical ideas, and OpenAI has launched their GPT Store, pulling the rug out from under a whole bunch of young AI startups building on top of their API.
If you’ve proven a product-market fit for a new “ChatGPT for X,” you may discover that you’ve essentially conducted free market research for a large incumbent company in that area, such as SalesForce, which can easily add a clone of your product to their portfolio. Alternatively, another startup might simply clone your idea, turning your product into an easily replaceable commodity, driving the price down, and making it challenging to establish a sustainable business.
So, what can a young AI startup do to defend itself against copycats attempting to clone its product?
Proprietaty Datasets
The ultimate strategic asset for an AI startup is to have exclusive access to a valuable and hard-to-copy dataset. In AI startups, the training data is often more valuable than the AI algorithms. For instance, OpenAI, despite its team of world-class AI experts, abandoned robotics because they lacked data.
The ultimate strategic asset for an AI startup is to have exclusive access to a valuable and hard-to-copy dataset.
A less potent version of this strategic asset is to have non-exclusive access to valuable data, such as open-source or public datasets, and then enrich it with additional insights. This can also involve aggregating multiple separate datasets into a unified whole, where the sum is greater than the parts. For example, while Salesforce lacks direct access to HubSpot’s data, an AI startup could aggregate these two data sources to uncover new insights for companies using both systems.
The problem with proprietary datasets is that they are often held by established incumbents, not emerging AI startups. For instance, Google possesses vast amounts of search data, and Tesla has an extensive collection of driving data. In contrast, an AI startup almost always begins with nothing unless it has an exclusive partnership with the owner of such a proprietary dataset.
Niche Specialization
My bet is that most early-stage startup opportunities lie in the application layer of AI, rather than the platform layer. OpenAI is rumored to have invested over $100 million in training GPT-4, indicating that competing at the platform level requires access to massive resources. An exception to this may be found in compliance-intensive domains, such as insurance or conducting business in the EU, where there might be opportunities in the platform layer for specialized or on-premises AI platform products.
While I believe that most opportunities lie in the application layer, pursuing horizontal products—those with broad applicability across various industries—poses a serious risk. You may find yourself merely conducting free market research for an incumbent, as horizontal product ideas often appeal to established companies, such as Microsoft, who may readily clone your idea and integrate it into their existing offerings.
A more effective strategy is to pursue an AI-powered idea specifically tailored to a particular niche—a vertical solution that delivers superior value to a targeted industry compared to any generic, horizontal product. Large players, such as Google or Amazon, often ignore verticals, and even if they do pursue them, they rarely allocate their A-players to these, for them, peripheral opportunities.
Avoid building AI for AI’s sake by solving problems that nobody cares about, even if they can be solved with AI.
Practical ideas for AI-powered startups within a niche or vertical include digitalizing workflows that were impossible to automate in the pre-AI era or developing applications that address pain points that are top priorities for executives in that vertical. Avoid building AI for AI’s sake by solving problems that nobody cares about, even if they can be solved with AI. Instead, focus on solving a problem that truly matters. In B2B startups, the litmus test is always whether someone is willing to pay for your product, rather than merely offering compliments and praise for your innovative idea. Don’t limit your solution to AI, but use whatever it takes, with AI being one tool in your toolbox.
A crucial benefit of the niche approach is that, over time, you can build a proprietary dataset within that vertical, which can be used for fine-tuning generic AI models. This strategy mirrors GitHub’s approach, utilizing its vast code repositories to fine-tune a generic GPT model for its Copilot AI assistant.
It’s important to acknowledge that niche specialization alone does not guarantee defensibility—at least, not until a proprietary dataset has been collected and utilized. This is because a copycat can just as easily attack a vertical product as a horizontal one. While focusing on a niche can reduce the number of competitors, it cannot safeguard against all potential rivals. For instance, you may have built the first “ChatGPT for X,” but then a copycat emerges, learns from your mistakes, and creates a superior version of “ChatGPT for X,” potentially drawing customers away from your original product.
Startup Velocity
A significant advantage that a startup holds over established players is its ability to move quickly. This applies not only to AI startups but to any startup. Until a proprietary dataset has been established to defend against copycats, the key is to rely on the advantage of speed—outpacing incumbents and other AI startups in the same domain. By prioritizing rapid development and delivering a solution that effectively addresses customer needs, startups can potentially enjoy the benefits of first-mover advantages, such as network effects.
Mathematically speaking, a startup cannot achieve instant defensibility. That is, something a small number of people can build in a short timeframe can be copied by competitors with similar or greater resources. If a compelling product-market fit exists, it would be a trivial task for Google to invest the same resources as the startup in pursuing this idea. However, it’s unlikely that they would be able to catch up with a fast-moving startup, especially one with a headstart.
In summary, my rule of thumb for defensibility in AI startups is to focus on a vertical, building a product that people within that niche love to use, and then outpace competitors in terms of innovation and speed to market. Over time, aim to accumulate and leverage a proprietary dataset within that niche, establishing long-term defensibility against copycats and other clones.